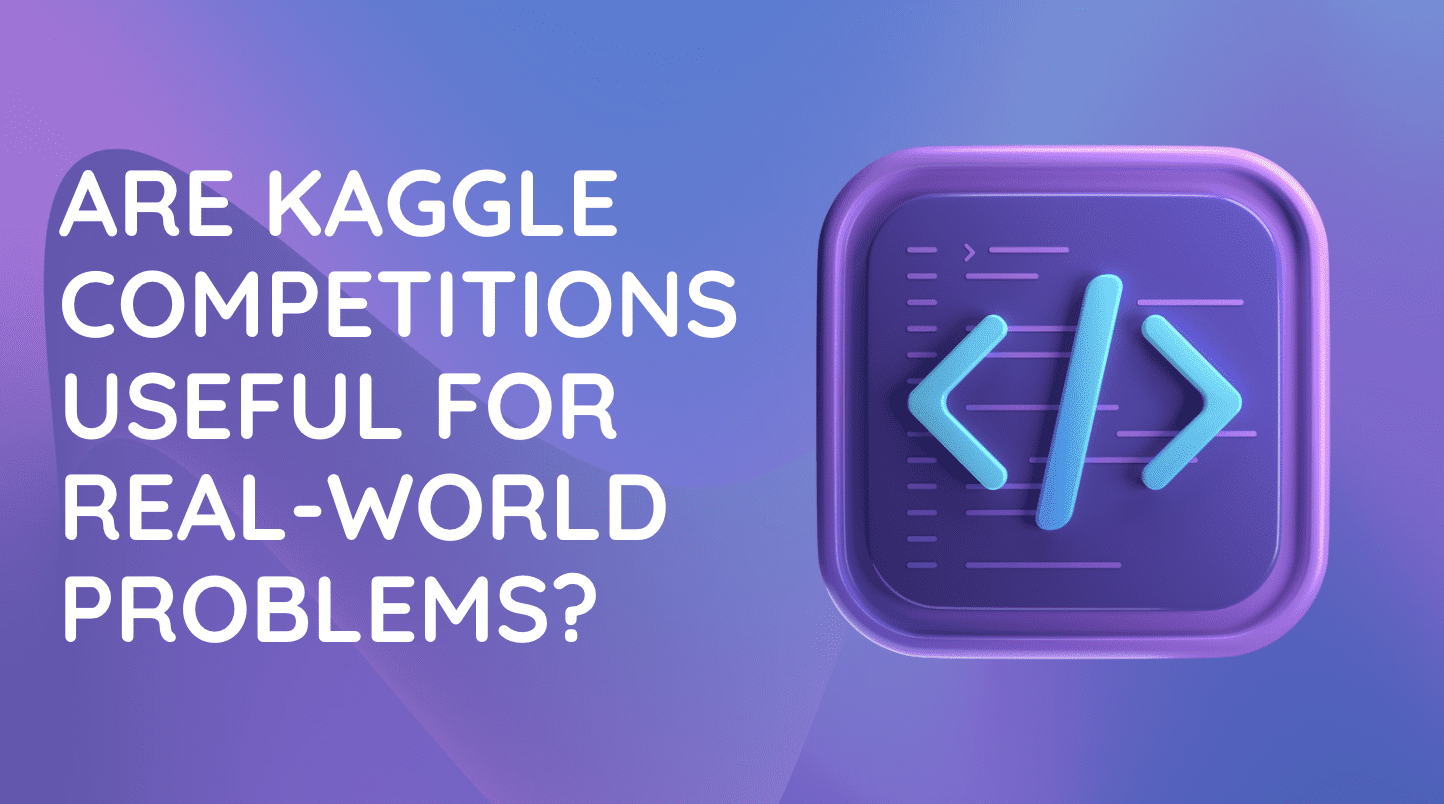
Image by Author
If you’re jumping into the tech industry or have been in it for a while, you would have heard of Kaggle. It is a data science competition platform which is aimed at data scientists and machine learning enthusiasts.
The online platform aims to guide users in their professional careers to reach their goals in their data science or machine learning journey with the powerful tools and resources it provides.
As people are trying to improve and progress in their careers, you will see a lot of people flock to online courses, competitions, and more. Kaggle is an amazing platform for people to test themselves, throw themselves in the deep end and come face to face with the reality of their skillset.
Many people have built projects on the Kaggle platform, having access to a variety of datasets, with great resources such as free access to NVIDIA K80 GPUs in kernels. The question we are going to pose today is ‘Are Kaggle Competitions Useful for Real-World Problems?’.
A question was raised on Quora: should I invest my time participating in Kaggle or working on interesting side projects? Which will be more beneficial for my career?
With a variety of responses, but as you can see in the image screenshot below explains the answer to your question.

Let’s get into whether Kaggle competitions are useful for real-world problems.
So we have spoken about how Kaggle competitions help your learning journey and how aspects of it reflect what happens in the real world. But is it useful for real-world problems? The overall answer is no. Let me explain why in different aspects.
Identifying the Problem
As a data scientist or machine learning engineer, your first task is to identify the problem or understand the current business problem that needs to be solved. For example, you may need to distinguish if the type of problem is supervised or unsupervised, decide which model you will use, etc.
This is one of the most important decisions you are going to make. If you don’t have an overall understanding of the organization, it will make your life harder as you cannot identify the root problem.
Real-world: Identify the problem or understand the current business problem that needs to be solved
Kaggle: You are provided with a detailed description of the problem and what you are evaluating.
Data Preparation
With Kaggle competitions, the host of the contest provides you with prepared datasets along with a detailed description of the problem at hand. This saves data scientists a lot of time going out to collect, clean and structure data – which happens in the real world.
Some believe that Kaggle spoon-fed new data scientists and machine learning engineers with provided data, allowing them to get straight to work. Data preparation is an important phase in the data science lifecycle, and Kaggle has shown to do it all for users.
In the real world, your company may or may not provide you with data. If they have not, you will have to collect it yourself, ensure it aligns with the problem at hand, and clean and structure it. You are also freely allowed to look for additional relevant data, whereas on Kaggle you are restricted to using outside data.
Real-world: Data collection and preparation help you to work around your identified problem.
Kaggle: Provides you with prepared data that is aligned with a detailed description of the problem at hand.
Feature Engineering
Once you have got your data and it’s all shiny clean, your next step as a data scientist is to go in and become a feature engineer. Feature engineering is rooted in your problem at hand, what you are trying to solve and how you are going to solve it.
With this, you will have a better understanding of how much time you will spend on feature engineering, and if other elements of the data science lifecycle are more important.
However, in Kaggle competitions, feature engineering plays a big role in where you end up on the leaderboard. Yes, feature engineering is part of the data science lifecycle, but real-world data science projects focus more on the factor that drives your model, rather than small incremental gains.
Real-world: The level of feature engineering is dependent on the problem at hand and where your focus is.
Kaggle: The level of feature engineering is used as an incentive to get higher up on the leaderboard.
Modelling
Choosing the correct model is based on a lot of factors, such as the explainability of the model, the data you are using, the performance of the model, and bringing the model to production. These are all in line with your problem at hand, as it is down to you to determine which one fits your business’s needs.
Whereas on Kaggle, users are more concerned about which model performs the best and processes the data they are working with. The factors that are taken into consideration when choosing their model are far less realistic than what is dealt with in the real world.
Real-world: Choosing the correct model based on a variety of factors that are linked to your business’s problem at hand.
Kaggle: Choosing the correct model based on performance as you are taking part in a competition.
Validation
Validation is an aspect that both Kaggle and the real world show resemblance. Validating the performance of your model is an important aspect as it allows you to explore where you can make changes to improve your model and shows you if your model has value in the real world.
Kaggle competitions show you how building a robust model is of use in the real world.
Model into Production
In the real world, the majority of models you are building are aimed to move into production. This is because there is a purpose behind your model, you were trying to solve a real-world problem. Your model will one way or another find its way to be integrated into the business process to help in future decision making.
On the other hand, when you’re taking part in a Kaggle competition, your #1 concern is where you ranked on the leaderboard and not how your model will be implemented and used in the future.
Real-world: Every model you build has a purpose and you want to move it into production to solve your business’s problem at hand.
Kaggle: The overall aim of building your model was to see where you ranked on the leaderboard and what you can do better next time in comparison to your competitors.
Kaggle teaches you a lot. Through Kaggle competitions and working on different tasks and datasets, you can learn a lot. Personally, I don’t believe there is any harm in learning more and coming across challenges. You just learn how to overcome these challenges by reflecting on your weaknesses and how to turn them into strengths.
Would you rather be in the position of knowing more before you land your dream job, or not knowing? The answer is pretty simple and it depends on what you want out of your career.
Kaggle competitions show you the performance of your model which is good for your learning journey. As stated in the screenshot above, you could assume that the performance of your model is really good, only to realize that it wasn’t as good as others in the same competition.
With that being said, Kaggle competitions push you during your learning journey, allowing you to compete with people from all over the world and up-skill as an individual.
In the real world, when you are working on projects you are given deadlines. Deadlines help you keep on top of your tasks which are in line with the organization’s business plan. Every deadline is the start of a new project.
Kaggle competitions have deadlines which reflect what your day-to-day tasks could typically look like. This is a great way to understand how your time is used as well as overcoming procrastination.
Based on the points we went over, the usefulness of Kaggle competitions is purely down to individuals. Yes, every aspect of a Kaggle competition may not mirror what happens in the real world, but many of us can say that about some of the things we learned at school.
Is that enough to say it is not useful for real-world problems?
Kaggle competitions provide you with a lot of learning experience and allow you to explore skills you may have never targeted before. There is a lot of experience that can come out of Kaggle competitions which can be used in your career later on.
Nisha Arya is a Data Scientist and Freelance Technical Writer. She is particularly interested in providing Data Science career advice or tutorials and theory based knowledge around Data Science. She also wishes to explore the different ways Artificial Intelligence is/can benefit the longevity of human life. A keen learner, seeking to broaden her tech knowledge and writing skills, whilst helping guide others.