AI Strategists are adept in building AI roadmap and vision for businesses. However, aligning the roadmap to the expected business outcomes becomes challenging, given the evolving scope of AI initiatives.

Image by Author
Hence, it is crucial to keep adapting and refining the AI strategy to ensure that it remains aligned with the evolving business objectives and technological landscape.
But before we start with the strategy itself, let’s discuss the role of an AI strategist.
AI strategists are fluent with AI workflows and map the business prerogatives to technical solutions leveraging AI. They comprehend the associated complexities along with opportunity estimation and do not necessarily need to know the intricacies of algorithms.
Let’s expand on these three pillars of opportunity estimation. Firstly, it is important to note that there are many innovative ways to solve a business problem, and not all of them require the use of sophisticated and advanced technology as that of AI.
Some can be easily solved by rules, while others can simply be automated and solve the problem to a reasonable extent.
Doing such an assessment is key to analyzing the baseline which involves taking an inventory stock of what part of the problem is solved with the existing solution. If the current solution is not acceptable, then the strategists make a trade-off by explaining the potential increase in the efficacy of the proposed AI-powered solution and the risks that come with it.

Image by Author
Primarily, the strategists ensure the team is AI-aware and opt for an advanced solution, fully cognizant of time, effort, cost, complexity, and the associated risks. It would be fair to say that an AI strategist is the linchpin to the success of AI transformation.
Equipped with strong business acumen, an AI strategist typically follows three factors to build a successful roadmap:
The first one is to ensure that the proposed solution is technically feasible. They identify the data requirements and evaluate whether the problem at hand warrants the use of AI. What if data is not available or is not authorized for model training or does not have accurate labels? All this falls under the purview of an AI strategist.
In addition to a feasible solution, the second aspect is viability. Even if it is possible to scale the solution, an AI strategist wears the techno-business lens to assess whether the proposed model development is financially viable for the business objectives. If the cost-benefit analysis suggests that the estimated benefit from the new AI/ML model development does not justify the non-trivial cost of building it, then it is best advised to drop the idea.
Any solution is good only if it provides value, which is often a challenge. Value could be in terms of a new revenue stream, a business differentiator, improved processes in the form of automation bringing efficiencies, and more. An AI strategist has a detailed methodical approach to defining the value proposition behind AI initiatives.
Phrases such as digital transformation or AI transformation might appear irrelevant in today’s rapidly evolving technological landscape.
One may ask that businesses need to be continuously innovating, leveraging emerging technologies, and adapting to market shifts. So, how do we define transformation when innovation is a continuous thing?
Let’s simplify and understand the core principles behind onboarding such a multi-year business evolution.
The transformation is, often, an inflection point when an organization realizes the need to revisit the legacy way of doing business. They understand that the status quo mode of operating a business is not sustainable, making them lose their competitive edge, thereby impacting their growth.

Image by Author
Hence, the rate at which they try the number of ideas accelerates rapidly and flows down to the funnel, making experiments work at scale. That is where the organization benefits from the compounded knowledge of an AI strategist over time, who has led multiple such AI transformations at scale. They are equipped with a toolkit consisting of adaptive frameworks, systems, and processes, which can be abstracted as strategies leading to successful AI transformation.
A few years ago, when the concept of AI strategy started becoming the focus of boardroom discussions, it caught everyone’s attention. Specifically, for the reason of having too many strategies – Business, AI, and Data.
It is easy to get confused amid multiple strategies around, such as business strategy, data strategy, and now, AI strategy. Here is how the three strategies work together in coherence.
Business strategy and vision are always at the top. It is crucial to have a clear business vision, the critical growth drivers, and a roadmap that aligns with the business objectives. Once the business leaders decide the “why and what”, next follows the “how”.
An AI strategist along with technologists focuses on the how part, to achieve the business vision through technology. It is important to note that technology is just an enabler. Hence, AI strategy is derived from business strategy, which means it requires extensive time to understand the business – the moat, and the competitive vantage point.
However, AI does not work on its own and needs data at its core to model the phenomenon. Hence, it works in tandem with data strategy.
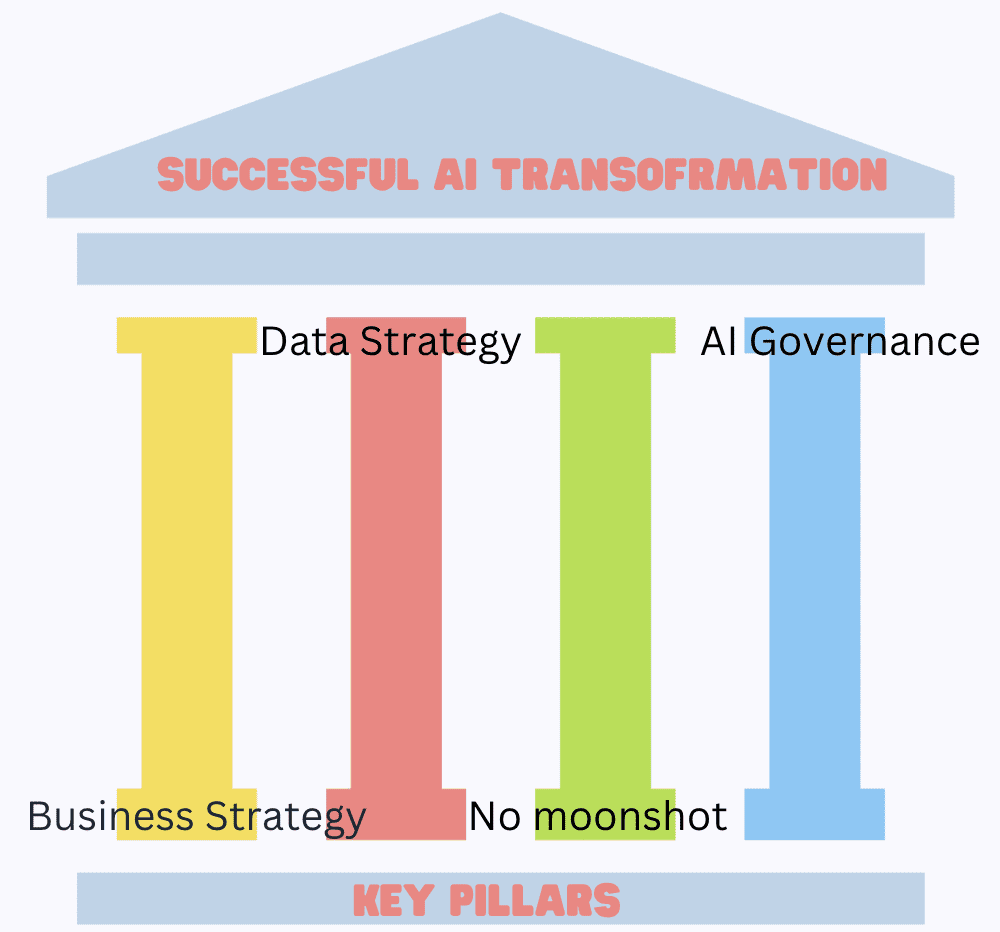
Image by Author
The next important aspect of designing a successful AI strategy is to ensure that AI teams do not give any moonshot commitment. This goes in line with the role of an AI strategist in assessing the feasibility of the proposed idea. AI projects bring a lot of “unknown unknowns” with them, hence it is essential to bake in foreseen and unforeseen risks.
The model is ready but is of no good use if it is not aligned with the responsible and ethical principles of building AI. Imagine having spent a huge amount of money building AI pipelines and workflows, data is in place and the predictions work just fine.
But only to realize that data has a bias, includes PII information, or a thing as basic but crucial as transparency and explainability.
It is important to note that predictions are of no good use until someone acts on them, and no one can act on predictions until they trust how and where they are coming from.
Hence, AI governance which includes extensive documentation on roles and responsibilities (ownership if it goes rogue), and the process of data collection, transformation, and training set, is the key driver of a successful implementation.
Understanding the trifecta of business, data, and AI strategy along with the key pillars for AI strategy are crucial to leading the organizations through a successful AI transformation.
Vidhi Chugh is an AI strategist and a digital transformation leader working at the intersection of product, sciences, and engineering to build scalable machine learning systems. She is an award-winning innovation leader, an author, and an international speaker. She is on a mission to democratize machine learning and break the jargon for everyone to be a part of this transformation.